What are the common challenges in business forecasting?
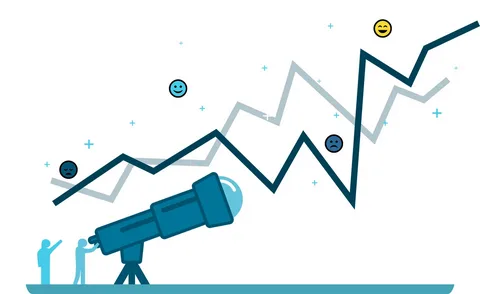
Business forecasting is an essential practice for organizations that seek to make informed decisions, manage risks, and achieve long-term growth. By predicting future trends, customer behavior, or market conditions, businesses can allocate resources more effectively and develop strategies that align with future opportunities. However, forecasting is not without its challenges. Several obstacles can undermine the accuracy and reliability of forecasts, and understanding these challenges is crucial for businesses aiming to enhance their forecasting efforts. Below are some of the most common challenges in business forecasting.
1. Data Quality Issues
Data serves as the foundation for business forecasting, but it is often riddled with issues that can impact forecast accuracy. Inaccurate, incomplete, outdated, or inconsistent data can lead to misleading predictions. This challenge is particularly prominent when organizations rely on historical data that may no longer reflect current market conditions or consumer preferences. Furthermore, data from various sources might not be compatible or may need to be cleaned and standardized before it can be used for forecasting. Poor data quality can result in faulty insights, undermining decision-making and strategy development.
2. Rapidly Changing Market Conditions
The business landscape is constantly evolving, and this can pose a significant challenge for forecasting. Rapid shifts in market dynamics, consumer behavior, technological advances, or regulatory changes can quickly render previous forecasts obsolete. For instance, during economic downturns or global crises (like the COVID-19 pandemic), the patterns and trends that were once reliable may no longer apply. In industries such as technology and fashion, where trends change quickly, the unpredictability of market conditions adds an additional layer of complexity to the forecasting process.
Forecasts that rely too heavily on historical data might fail to account for the new variables that come with these changing conditions, leaving businesses unprepared for sudden shifts in the market.
3. Selecting the Appropriate Forecasting Model
There are various forecasting models available, each with its strengths and weaknesses. Selecting the right one depends on the nature of the data, the business's specific needs, and the forecasting horizon. Common models include time series analysis, causal models, machine learning algorithms, and judgmental forecasting. Each model comes with its own set of assumptions and limitations, and choosing the wrong model can result in inaccurate predictions.
Time series forecasting, for example, is highly effective when there are clear historical patterns to follow. However, if future conditions diverge significantly from historical trends, this method may lead to errors. Causal models, on the other hand, attempt to identify relationships between variables but can be overly sensitive to assumptions. Machine learning models can accommodate complex and large datasets, but they often require significant computational power and expert knowledge to apply effectively.
A business's specific context and goals must guide the model selection process. However, even the best model is only as good as the data it works with, meaning that no model can completely overcome poor-quality data.
4. Lack of Skilled Forecasters
Effective forecasting is a complex task that requires both technical expertise and experience. A lack of skilled professionals who understand forecasting methods and can interpret the results accurately may hinder the forecasting process. Business leaders often assume that forecasting can be done by anyone with basic statistical knowledge, but in reality, it demands a deep understanding of the underlying factors that drive business performance and market dynamics.
Without skilled forecasters, businesses might struggle to build or implement effective forecasting systems. This gap in expertise can also lead to overreliance on automated systems, which can produce misleading forecasts without human intervention to provide context or judgment.
5. External Factors and Uncertainty
Forecasting is inherently uncertain, especially when external factors are at play. Unpredictable events such as natural disasters, geopolitical tensions, or unexpected economic shifts can have a significant impact on forecasts. No model can account for every possible external event, making it essential for businesses to prepare for uncertainty and develop contingency plans.
External factors, while impossible to predict with complete certainty, should be monitored continuously to update forecasts and adjust strategies as needed. Being aware of these potential disruptions and incorporating flexibility into forecasting processes can help mitigate their impact.
6. Over-Reliance on Historical Data
While historical data is a valuable tool in forecasting, relying too heavily on it can be problematic. In certain industries, past trends may no longer be relevant due to shifts in technology, consumer preferences, or competitive forces. For example, a retail company that historically relied on seasonal sales patterns might be caught off guard if consumer behavior changes dramatically due to a new trend or external event.
Forecasting models that depend on historical data alone may fail to recognize emerging patterns or disruptions in the market. Therefore, it is crucial to supplement historical data with insights from current trends, competitor actions, and expert opinions to improve forecast accuracy.
7. Time and Resource Constraints
Forecasting can be a time-consuming and resource-intensive process. Collecting and analyzing large amounts of data, selecting the appropriate model, and refining forecasts to incorporate new information requires substantial effort and investment. For smaller businesses or those with limited resources, it may be difficult to allocate the necessary time and staff to ensure that forecasting is conducted effectively.
In such cases, businesses may resort to simplified forecasting methods or rely on third-party services, but these solutions may come with their own limitations in terms of accuracy and customization.
8. Bias and Human Judgment
Even with advanced forecasting models, human judgment often plays a role in interpreting and adjusting the results. However, human biases can affect forecasting accuracy. Cognitive biases such as overconfidence, confirmation bias, or anchoring can influence the way forecasters interpret data or make decisions. These biases can lead to overoptimistic predictions or failure to account for significant risks, ultimately distorting the forecast and leading to poor business decisions.
Conclusion
Business forecasting is a critical tool for organizations seeking to make data-driven decisions, but it comes with its share of challenges. Data quality issues, rapidly changing market conditions, and difficulties in selecting the right forecasting model all contribute to the complexity of the forecasting process. Additionally, businesses must be mindful of external uncertainties, resource limitations, and the potential for human bias in interpreting forecasts. By recognizing these challenges and proactively addressing them, companies can enhance the accuracy and reliability of their forecasts, helping them navigate the uncertain future more effectively.
- Arts
- Business
- Computers
- Games
- Health
- Home
- Kids and Teens
- Money
- News
- Recreation
- Reference
- Regional
- Science
- Shopping
- Society
- Sports
- Бизнес
- Деньги
- Дом
- Досуг
- Здоровье
- Игры
- Искусство
- Источники информации
- Компьютеры
- Наука
- Новости и СМИ
- Общество
- Покупки
- Спорт
- Страны и регионы
- World